Tag Archive: Deep
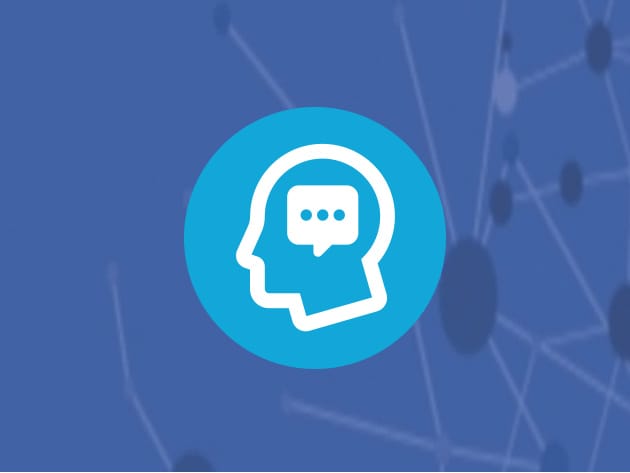
Pay What You Want: AI & Deep Learning Bundle for $1
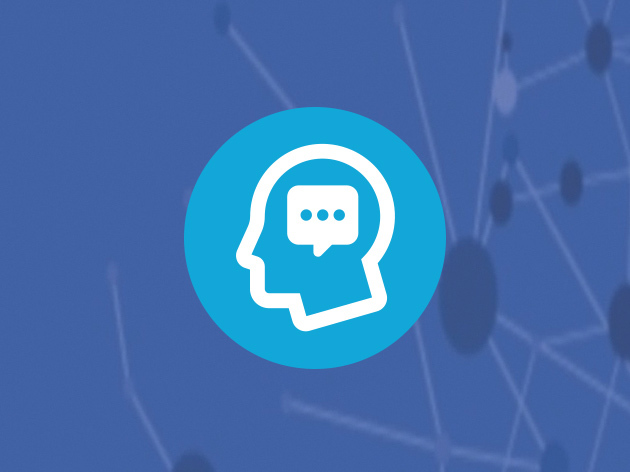
KEY FEATURES
You’ve seen deep learning everywhere, but you may not have realized it. This discipline is one of the leading solutions for image recognition, speech recognition, object recognition, and language translation – basically the tools you see Google roll out every day. Over this course, you’ll use Python to expand your deep learning knowledge to cover backpropagation and its ability to train neural networks.
- Access 19 lectures & 2 hours of content 24/7
- Train neural networks in deep learning & to understand automatic differentiation
- Cover convolutional & recurrent neural networks
- Build up the theory that covers supervised learning
- Integrate search & image recognition, & object processing
- Examine the performance of the sentimental analysis model
PRODUCT SPECS
Details & Requirements
- Length of time users can access this course: lifetime
- Access options: web streaming, mobile streaming
- Certification of completion not included
- Redemption deadline: redeem your code within 30 days of purchase
- Experience level required: all levels
Compatibility
- Internet required
THE EXPERT
Eder Santana is a PhD candidate in Electrical and Computer Engineering. His thesis topic is on Deep and Recurrent neural networks. After working for 3 years with Kernel Machines (SVMs, Information Theoretic Learning, and so on), Eder moved to the field of deep learning 2.5 years ago, when he started learning Theano, Caffe, and other machine learning frameworks. Now, Eder contributes to Keras: Deep Learning Library for Python. Besides deep learning, he also likes data visualization and teaching machine learning, either on online forums or as a teacher assistant.
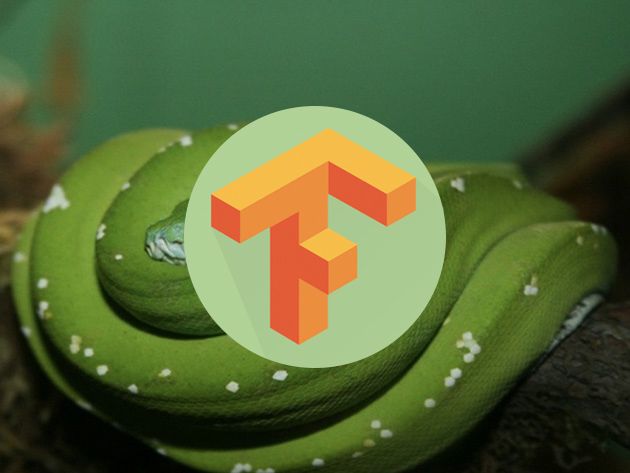
KEY FEATURES
Deep learning is the intersection of statistics, artificial intelligence, and data to build accurate models, and is one of the most important new frontiers in technology. TensorFlow is one of the newest and most comprehensive libraries for implementing deep learning. Over this course you’ll explore some of the possibilities of deep learning, and how to use TensorFlow to process data more effectively than ever.
- Access 22 lectures & 2 hours of content 24/7
- Discover the efficiency & simplicity of TensorFlow
- Process & change how you look at data
- Sift for hidden layers of abstraction using raw data
- Train your machine to craft new features to make sense of deeper layers of data
- Explore logistic regression, convolutional neural networks, recurrent neural networks, high level interfaces, & more
PRODUCT SPECS
Details & Requirements
- Length of time users can access this course: lifetime
- Access options: web streaming, mobile streaming
- Certification of completion not included
- Redemption deadline: redeem your code within 30 days of purchase
- Experience level required: all levels
Compatibility
- Internet required
THE EXPERT
Dan Van Boxel is a Data Scientist and Machine Learning Engineer with over 10 years of experience. He is most well-known for “Dan Does Data,” a YouTube livestream demonstrating the power and pitfalls of neural networks. He has developed and applied novel statistical models of machine learning to topics such as accounting for truck traffic on highways, travel time outlier detection, and other areas. Dan has also published research and presented findings at the Transportation Research Board and other academic journals.
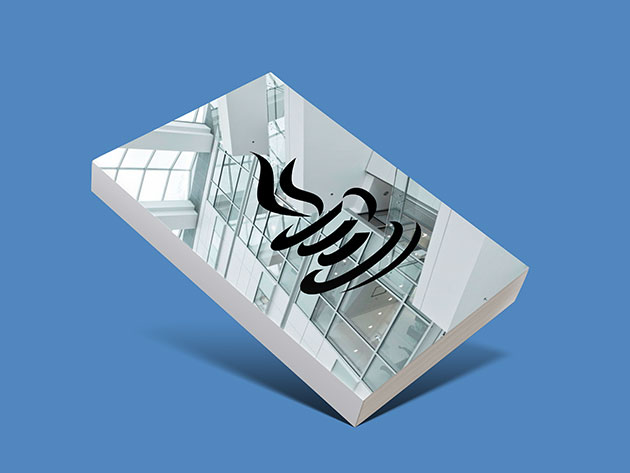
KEY FEATURES
AI and Deep Learning are transforming the way we understand software, making computers more intelligent than we could even imagine just a decade ago. Starting with an introduction to basic machine learning algorithms, this course takes you further into this vital world of stunning predictive insights and remarkable machine intelligence.
- Access 254 pages of content 24/7
- Get a practical deep dive into machine learning & deep learning algorithms
- Implement machine learning algorithms related to deep learning
- Explore neural networks using some of the most popular Deep Learning frameworks
- Dive into Deep Belief Nets & Stacked Denoising Autoencoders algorithms
- Discover more deep learning algorithms w/ Dropout & Convolutional Neural Networks
- Gain an insight into the deep learning library DL4J & its practical uses
- Get to know device strategies to use deep learning algorithms & libraries in the real world
- Explore deep learning further w/ Theano & Caffe
PRODUCT SPECS
Details & Requirements
- Length of time users can access this course: lifetime
- Access options: web streaming, mobile streaming
- Certification of completion not included
- Redemption deadline: redeem your code within 30 days of purchase
- Experience level required: all levels
Compatibility
- Internet required
THE EXPERT
Packt’s mission is to help the world put software to work in new ways, through the delivery of effective learning and information services to IT professionals. Working towards that vision, it has published over 3,000 books and videos so far, providing IT professionals with the actionable knowledge they need to get the job done–whether that’s specific learning on an emerging technology or optimizing key skills in more established tools.
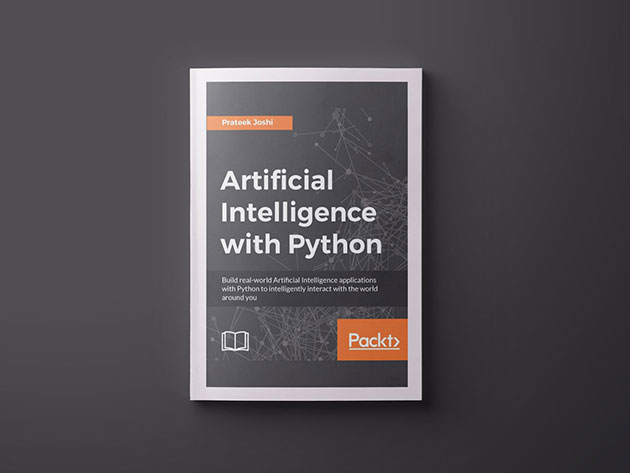
KEY FEATURES
Artificial Intelligence is especially relevant in today’s technology and data-driven world. It’s used in search engines, image recognition, robotics, finance, and many other industries. In this book, you’ll explore various real-world scenarios while learning about various algorithms that can be used to build AI applications. Starting out from the basics of AI, you’ll learn how to develop various building blocks using different data mining techniques before delving into more advanced subjects.
- Access 446 pages of digital content 24/7
- Explore different classification & regression techniques
- Understand the concept of clustering & how to use it to automatically segment data
- See how to build an intelligent recommender system
- Discover logic programming & how to use it
- Build automatic speech recognition systems
- Understand the basics of heuristic search & genetic programming
- Develop games using Artificial Intelligence
- Learn how reinforcement learning works
- Discover how to build intelligent applications centered on images, text, & time series data
- See how to use deep learning algorithms & build applications based on them
PRODUCT SPECS
Details & Requirements
- Length of time users can access this course: lifetime
- Access options: web streaming, mobile streaming
- Certification of completion not included
- Redemption deadline: redeem your code within 30 days of purchase
- Experience level required: all levels
Compatibility
- Internet required
THE EXPERT
Packt’s mission is to help the world put software to work in new ways, through the delivery of effective learning and information services to IT professionals. Working towards that vision, it has published over 4,000 books and videos so far, providing IT professionals with the actionable knowledge they need to get the job done–whether that’s specific learning on an emerging technology or optimizing key skills in more established tools.
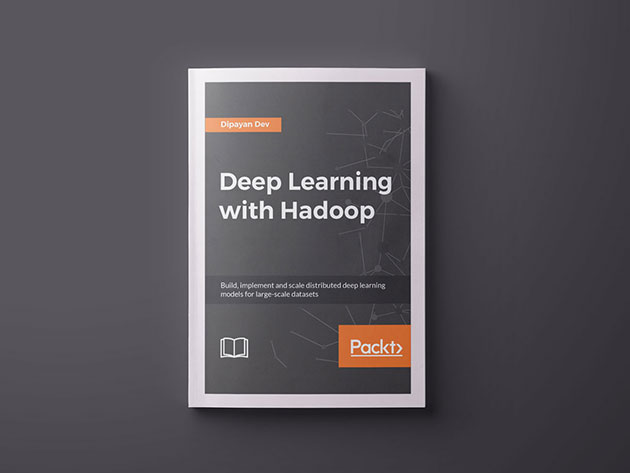
KEY FEATURES
This book will teach you how to deploy large-scale datasets in deep neural networks with Hadoop for optimal performance. Starting with an introduction to deep learning, you’ll learn how to set up a Hadoop envrionment and implement a variety of deep learning models. By the end of the book, you’ll know how to deploy various deep neural networks in distributed systems using Hadoop.
- Access 206 pages of digital content 24/7
- Explore deep learning & various models associated w/ it
- Understand the challenges of implementing distributed deep learning w/ Hadoop & how to overcome it
- Implement Convolutional Neural Network (CNN) w/ deeplearning4j
- Delve into the implementation of Restricted Boltzmann Machines (RBM)
- Understand the mathematical explanation for implement Recurrent Neural Networks (RNN)
- Get hands on practice w/ deep learning with Hadoop
PRODUCT SPECS
- Length of time users can access this course: lifetime
- Access options: web streaming, mobile streaming
- Certification of completion not included
- Redemption deadline: redeem your code within 30 days of purchase
- Experience level required: all levels
Compatibility
- Internet required
THE EXPERT
Packt’s mission is to help the world put software to work in new ways, through the delivery of effective learning and information services to IT professionals. Working towards that vision, it has published over 4,000 books and videos so far, providing IT professionals with the actionable knowledge they need to get the job done–whether that’s specific learning on an emerging technology or optimizing key skills in more established tools.
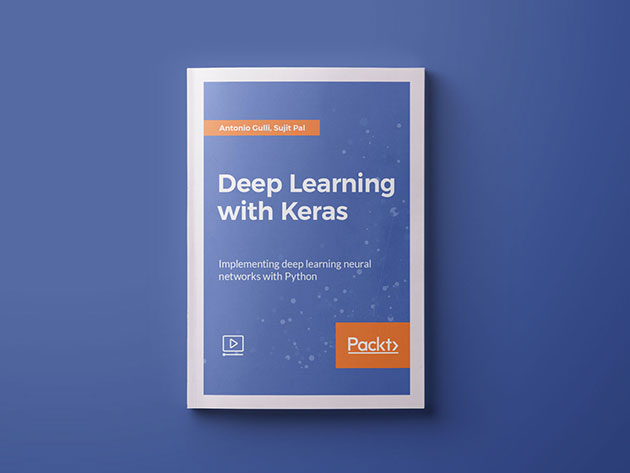
KEY FEATURES
This book starts by introducing you to supervised learning algorithms such as simple linear regression, the classical multilayer peceptron, and more sophisticated deep convolutional networks. You’ll also explore image processing, Recurrent Networks, and unsupervised learning algorithms such as Autoencoders. Finally, you’ll take a look at Reinforcement Learning and its application to AI game playing, another popular direction of research and application of neural networks.
- Access 318 pages of digital content 24/7
- Optimize step-by-step functions on a large neural network using the Backpropagation Algorithm
- Fine tune a neural network to improve the quality of results
- Use deep learning for image & audio processing
- Utilize Recursive Neural Tensor Networks (RNTNs) to outperform standard word embedding in special cases
- Identify problems for which Recurrent Neural Network (RNN) solutions are suitable
- Explore the process required to implement Autoencoders
- Evolve a deep neural network using reinforcement learning
PRODUCT SPECS
Details & Requirements
- Length of time users can access this course: lifetime
- Access options: web streaming, mobile streaming
- Certification of completion not included
- Redemption deadline: redeem your code within 30 days of purchase
- Experience level required: all levels
Compatibility
- Internet required
THE EXPERT
Packt’s mission is to help the world put software to work in new ways, through the delivery of effective learning and information services to IT professionals. Working towards that vision, it has published over 4,000 books and videos so far, providing IT professionals with the actionable knowledge they need to get the job done–whether that’s specific learning on an emerging technology or optimizing key skills in more established tools.
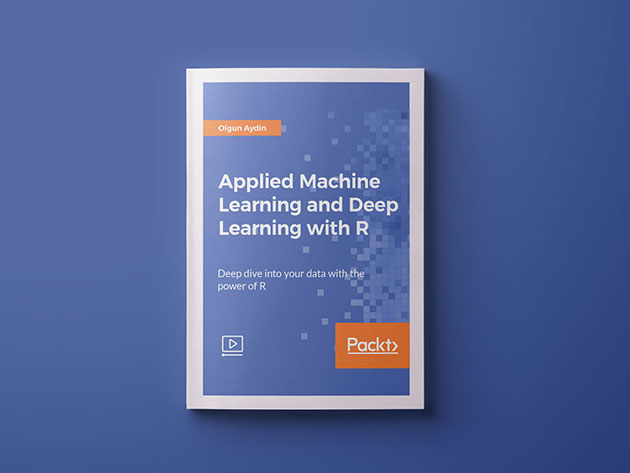
KEY FEATURES
In this course, you’ll examine in detail the R programming language, the most popular statistical programming language in the world today. You’ll start by exploring different learning methods, clustering, classification, model evaluation methods and performance metrics. As you progress to more advanced subjects, you’ll develop the skills necessary to perform a variety of tasks with R.
- Access 35 lectures & 4 hours of content 24/7
- Delve into the general structure of clustering algorithms
- Develop applications in the R environment by using clustering & classification algorithms for real-life problems
- Use general definitions about artificial neural networks
- Explore the elements of deep learning neural networks & other types of deep learning networks
- Dive into developing machine learning algorithms w/ SparkR
PRODUCT SPECS
Details & Requirements
- Length of time users can access this course: lifetime
- Access options: web streaming, mobile streaming
- Certification of completion not included
- Redemption deadline: redeem your code within 30 days of purchase
- Experience level required: all levels
Compatibility
- Internet required
THE EXPERT
Olgun is PhD candidate at Department of Statistics, Mimar Sinan University. He has been working on Deep Learning for his PhD thesis. Also working as Data Scientist.He is so familiar with Big Data technologies like Hadoop, Spark and able to use Hive, Impala. He is a big fan of R. Also he really loves to work with Shiny, SparkR.He has many academic papers and proceedings about applications of statistics on different disciplines. Mr. Olgun really loves statistic and loves to investigate new methods, share his experience with people.
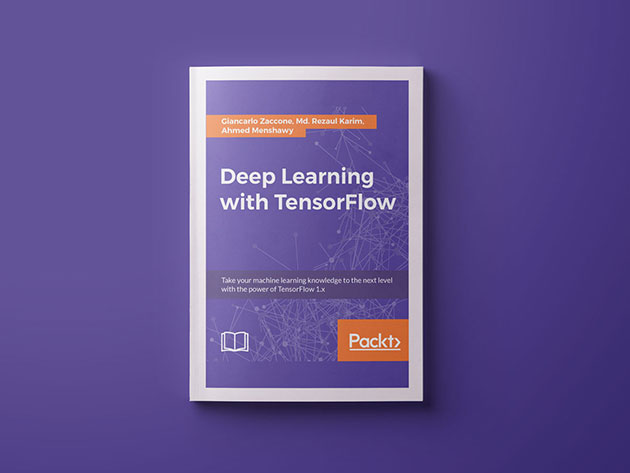
KEY FEATURES
Deep learning is the step that comes after machine learning, and has more advanced implementations. Throughout this book, you’ll learn how to implement deep learning algorithms for machine learning systems and integrate them into your product offerings, including search, image recognition, and language processing. After finishing the book, you’ll be familiar with machine learning techniques, in particular the use of TensorFlow for deep learning.
- Access 320 pages of digital content 24/7
- Learn about machine learning landscapes along w/ the historical development & progress of deep learning
- Discuss deep machine intelligence & GPU computing w/ the latest TensorFlow 1.x
- Access public datasets & utilize them using TensorFlow to load, process, and transform data
- Use TensorFlow on real-world datasets, including images, text, & more
- Learn how to evaluate the performance of your deep learning models
- Use deep learning for scalable object detection & mobile computing
- Train machines quickly to learn from data by exploring reinforcement learning techniques
- Explore active areas of deep learning research & applications
PRODUCT SPECS
Details & Requirements
- Length of time users can access this course: lifetime
- Access options: web streaming, mobile streaming
- Certification of completion not included
- Redemption deadline: redeem your code within 30 days of purchase
- Experience level required: all levels
Compatibility
- Internet required
THE EXPERT
Packt’s mission is to help the world put software to work in new ways, through the delivery of effective learning and information services to IT professionals. Working towards that vision, it has published over 4,000 books and videos so far, providing IT professionals with the actionable knowledge they need to get the job done–whether that’s specific learning on an emerging technology or optimizing key skills in more established tools.
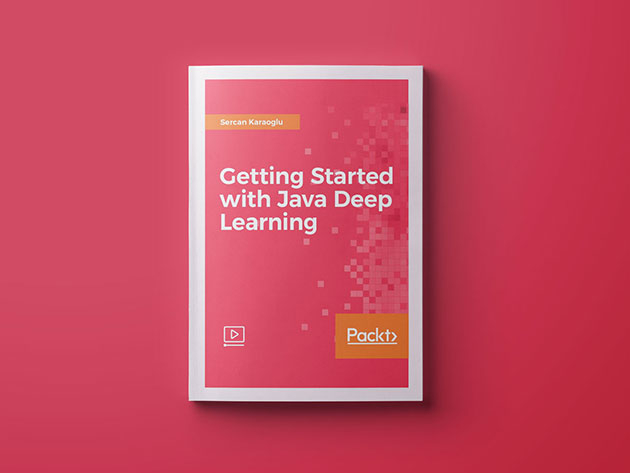
KEY FEATURES
AI and deep learning are transforming the way we understand software, making computers more intelligent than we could imagine even a decade ago. Deep learning algorithms are being used across a broad range of industries to produce hardware like self-driving cars, personal assistant computers, and decision support systems. As the fundamental driver of AI, Java is becoming a more vital and valuable skill in the global economy, and this course will introduce you to using Java for deep learning.
- Access 20 lectures & 2 hours of content 24/7
- Install the environment precisely
- Use the DL4J & apply deep learning to a range of real-world use cases
- Get an introduction to neural networks & implementing them
- Learn about various deep learning algorithms
- Tune Apache Spark
PRODUCT SPECS
Details & Requirements
- Length of time users can access this course: lifetime
- Access options: web streaming, mobile streaming
- Certification of completion not included
- Redemption deadline: redeem your code within 30 days of purchase
- Experience level required: all levels
Compatibility
- Internet required
THE EXPERT
Sercan Karaoglu gained his BSc in Mathematics Engineering at Istanbul Technical University. Karaoglu also completed a Research and Development project at age 23, at Foreks, in collaboration with The Scientific and Technological Research Council of Turkey(TUBITAK). This project was related to the application of Artificial Neural Networks in Financial Trading Decision Support Systems and Market Simulation for Intraday and Daily Trading.
Currently, he develops High Throughput-Low Latency Reactive Microservices and Reactive Stream applications at work and researches the topics of Deep Learning and Machine Learning. He is Java Software Engineer at the Dissemination Department of Foreks Information Systems, which is one of the leading IT companies in Turkey’s financial sector. It has specialized in software that is directly integrated with financial professionals and Istanbul Stock Market for over 26 years.
He is currently studying for his MSc in Computer Engineering at Bahcesehir University in the field of Big Data Analytics and Management.
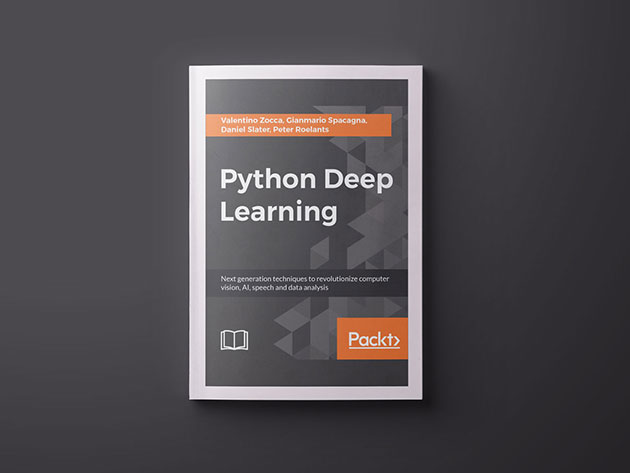
KEY FEATURES
With increasing interest in AI around the world, deep learning has attracted a great deal of public attention. Every day, deep learning algorithms are used broadly across different industries. This book will give you a practical introduction to AI, including best practices using real-world use cases. You’ll learn to recognize and extract information to increase predictive accuracy and optimize results.
- Access 406 pages of digital content 24/7
- Get a practical deep dive into deep learning algorithms
- Explore deep learning further with Theano, Caffe, Keras, & TensorFlow
- Learn about two of the most powerful techniques at the core of many practical deep learning implementations: Auto-Encoders & Restricted Boltzmann Machines
- Dive into Deep Belief Nets & Deep Neural Networks
- Discover more deep learning algorithms with Dropout & Convolutional Neural Networks
- Get to know device strategies so you can use deep learning algorithms & libraries in the real world
PRODUCT SPECS
Details & Requirements
- Length of time users can access this course: lifetime
- Access options: web streaming, mobile streaming
- Certification of completion not included
- Redemption deadline: redeem your code within 30 days of purchase
- Experience level required: all levels
Compatibility
- Internet required
THE EXPERT
Packt’s mission is to help the world put software to work in new ways, through the delivery of effective learning and information services to IT professionals. Working towards that vision, it has published over 4,000 books and videos so far, providing IT professionals with the actionable knowledge they need to get the job done–whether that’s specific learning on an emerging technology or optimizing key skills in more established tools.
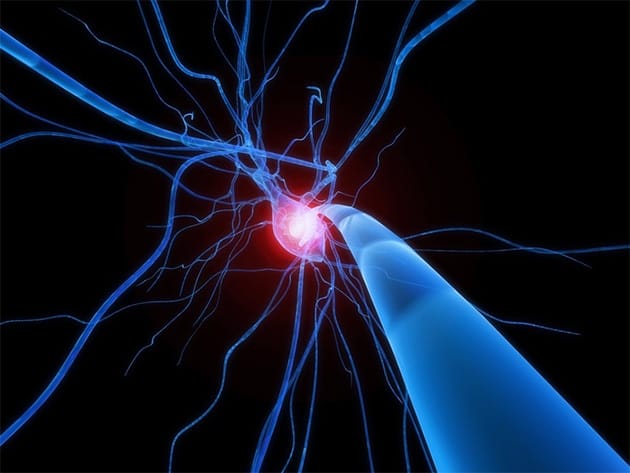
The AI and Deep Machine Learning Bundle for $39
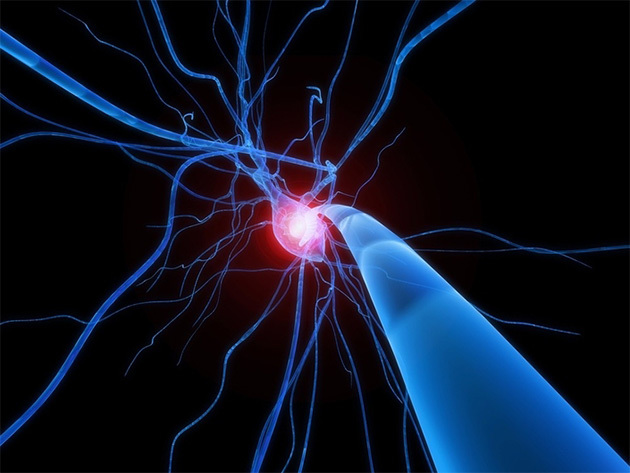
KEY FEATURES
Deep Learning is a set of powerful algorithms that are the force behind self-driving cars, image searching, voice recognition, and many, many more applications we consider decidedly “futuristic.” One of the central foundations of deep learning is linear regression; using probability theory to gain deeper insight into the “line of best fit.” This is the first step to building machines that, in effect, act like neurons in a neural network as they learn while they’re fed more information. In this course, you’ll start with the basics of building a linear regression module in Python, and progress into practical machine learning issues that will provide the foundations for an exploration of Deep Learning.
- Access 20 lectures & 2 hours of content 24/7
- Use a 1-D linear regression to prove Moore’s Law
- Learn how to create a machine learning model that can learn from multiple inputs
- Apply multi-dimensional linear regression to predict a patient’s systolic blood pressure given their age & weight
- Discuss generalization, overfitting, train-test splits, & other issues that may arise while performing data analysis
Like what you’re learning? Try out the The Advanced Guide to Deep Learning and Artificial Intelligence next.
PRODUCT SPECS
Details & Requirements
- Length of time users can access this course: lifetime
- Access options: web streaming, mobile streaming
- Certification of completion not included
- Redemption deadline: redeem your code within 30 days of purchase
- Experience level required: all levels, but you must have some knowledge of calculus, linear algebra, probability, Python, and Numpy
- All code for this course is available for download here, in the directory linear_regression_class
Compatibility
- Internet required
THE EXPERT
The Lazy Programmer is a data scientist, big data engineer, and full stack software engineer. For his master’s thesis he worked on brain-computer interfaces using machine learning. These assist non-verbal and non-mobile persons to communicate with their family and caregivers.
He has worked in online advertising and digital media as both a data scientist and big data engineer, and built various high-throughput web services around said data. He has created new big data pipelines using Hadoop/Pig/MapReduce, and created machine learning models to predict click-through rate, news feed recommender systems using linear regression, Bayesian Bandits, and collaborative filtering and validated the results using A/B testing.
He has taught undergraduate and graduate students in data science, statistics, machine learning, algorithms, calculus, computer graphics, and physics for students attending universities such as Columbia University, NYU, Humber College, and The New School.
Multiple businesses have benefitted from his web programming expertise. He does all the backend (server), frontend (HTML/JS/CSS), and operations/deployment work. Some of the technologies he has used are: Python, Ruby/Rails, PHP, Bootstrap, jQuery (Javascript), Backbone, and Angular. For storage/databases he has used MySQL, Postgres, Redis, MongoDB, and more.
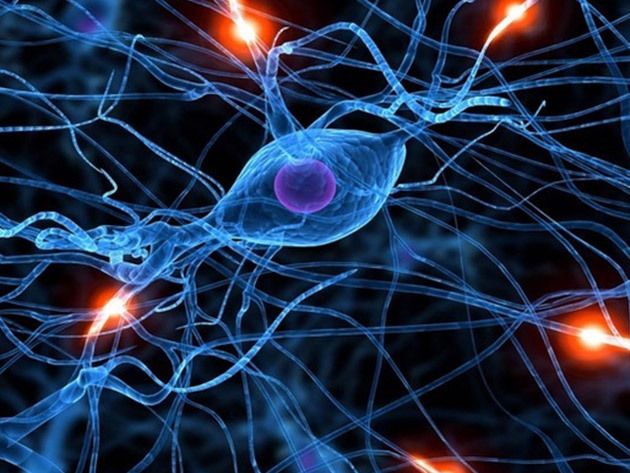
KEY FEATURES
Logistic regression is one of the most fundamental techniques used in machine learning, data science, and statistics, as it may be used to create a classification or labeling algorithm that quite resembles a biological neuron. Logistic regression units, by extension, are the basic bricks in the neural network, the central architecture in deep learning. In this course, you’ll come to terms with logistic regression using practical, real-world examples to fully appreciate the vast applications of Deep Learning.
- Access 31 lectures & 3 hours of content 24/7
- Code your own logistic regression module in Python
- Complete a course project that predicts user actions on a website given user data
- Use Deep Learning for facial expression recognition
- Understand how to make data-driven decisions
Like what you’re learning? Try out the The Advanced Guide to Deep Learning and Artificial Intelligence next.
PRODUCT SPECS
Details & Requirements
- Length of time users can access this course: lifetime
- Access options: web streaming, mobile streaming
- Certification of completion not included
- Redemption deadline: redeem your code within 30 days of purchase
- Experience level required: all levels, but you must have some knowledge of calculus, linear algebra, probability, Python, and Numpy
- All code for this course is available for download here, in the directory logistic_regression_class
Compatibility
- Internet required
THE EXPERT
The Lazy Programmer is a data scientist, big data engineer, and full stack software engineer. For his master’s thesis he worked on brain-computer interfaces using machine learning. These assist non-verbal and non-mobile persons to communicate with their family and caregivers.
He has worked in online advertising and digital media as both a data scientist and big data engineer, and built various high-throughput web services around said data. He has created new big data pipelines using Hadoop/Pig/MapReduce, and created machine learning models to predict click-through rate, news feed recommender systems using linear regression, Bayesian Bandits, and collaborative filtering and validated the results using A/B testing.
He has taught undergraduate and graduate students in data science, statistics, machine learning, algorithms, calculus, computer graphics, and physics for students attending universities such as Columbia University, NYU, Humber College, and The New School.
Multiple businesses have benefitted from his web programming expertise. He does all the backend (server), frontend (HTML/JS/CSS), and operations/deployment work. Some of the technologies he has used are: Python, Ruby/Rails, PHP, Bootstrap, jQuery (Javascript), Backbone, and Angular. For storage/databases he has used MySQL, Postgres, Redis, MongoDB, and more.
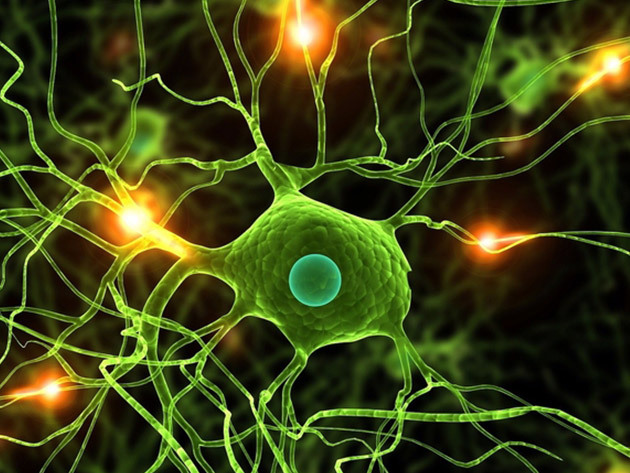
KEY FEATURES
Artificial neural networks are the architecture that make Apple’s Siri recognize your voice, Tesla’s self-driving cars know where to turn, Google Translate learn new languages, and so many more technological features you have quite possibly taken for granted. The data science that unites all of them is Deep Learning. In this course, you’ll build your very first neural network, going beyond basic models to build networks that automatically learn features.
- Access 37 lectures & 4 hours of content 24/7
- Extend the binary classification model to multiple classes uing the softmax function
- Code the important training method, backpropagation, in Numpy
- Implement a neural network using Google’s TensorFlow library
- Predict user actions on a website given user data using a neural network
- Use Deep Learning for facial expression recognition
- Learn some of the newest development in neural networks
Like what you’re learning? Try out the The Advanced Guide to Deep Learning and Artificial Intelligence next.
PRODUCT SPECS
Details & Requirements
- Length of time users can access this course: lifetime
- Access options: web streaming, mobile streaming
- Certification of completion not included
- Redemption deadline: redeem your code within 30 days of purchase
- Experience level required: intermediate, but you must have some knowledge of calculus, linear algebra, probability, Python, and Numpy
- All code for this course is available for download here, in the directory ann_class
Compatibility
- Internet required
THE EXPERT
The Lazy Programmer is a data scientist, big data engineer, and full stack software engineer. For his master’s thesis he worked on brain-computer interfaces using machine learning. These assist non-verbal and non-mobile persons to communicate with their family and caregivers.
He has worked in online advertising and digital media as both a data scientist and big data engineer, and built various high-throughput web services around said data. He has created new big data pipelines using Hadoop/Pig/MapReduce, and created machine learning models to predict click-through rate, news feed recommender systems using linear regression, Bayesian Bandits, and collaborative filtering and validated the results using A/B testing.
He has taught undergraduate and graduate students in data science, statistics, machine learning, algorithms, calculus, computer graphics, and physics for students attending universities such as Columbia University, NYU, Humber College, and The New School.
Multiple businesses have benefitted from his web programming expertise. He does all the backend (server), frontend (HTML/JS/CSS), and operations/deployment work. Some of the technologies he has used are: Python, Ruby/Rails, PHP, Bootstrap, jQuery (Javascript), Backbone, and Angular. For storage/databases he has used MySQL, Postgres, Redis, MongoDB, and more.
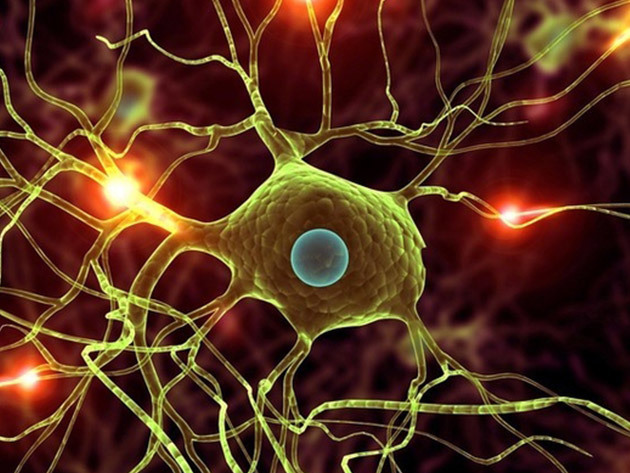
KEY FEATURES
The applications of Deep Learning are many, and constantly growing, just like the neural networks that it supports. In this course, you’ll delve into advanced concepts of Deep Learning, starting with the basics of TensorFlow and Theano, understanding how to build neural networks with these popular tools. Using these tools, you’ll learn how to build and understand a neural network, knowing exactly how to visualize what is happening within a model as it learns.
- Access 23 lectures & 3 hours of programming 24/7
- Discover batch & stochastic gradient descent, two techniques that allow you to train on a small sample of data at each iteration, greatly speeding up training time
- Discuss how momentum can carry you through local minima
- Learn adaptive learning rate techniques like AdaGrad & RMSprop
- Explore dropout regularization & other modern neural network techniques
- Understand the variables & expressions of TensorFlow & Theano
- Set up a GPU-instance on AWS & compare the speed of CPU vs GPU for training a deep neural network
- Look at the MNIST dataset & compare against known benchmarks
Like what you’re learning? Try out the The Advanced Guide to Deep Learning and Artificial Intelligence next.
PRODUCT SPECS
Details & Requirements
- Length of time users can access this course: lifetime
- Access options: web streaming, mobile streaming
- Certification of completion not included
- Redemption deadline: redeem your code within 30 days of purchase
- Experience level required: all levels, but you must have some knowledge of calculus, linear algebra, probability, Python, and Numpy
- All code for this course is available for download here, in the directory ann_class2
Compatibility
- Internet required
THE EXPERT
The Lazy Programmer is a data scientist, big data engineer, and full stack software engineer. For his master’s thesis he worked on brain-computer interfaces using machine learning. These assist non-verbal and non-mobile persons to communicate with their family and caregivers.
He has worked in online advertising and digital media as both a data scientist and big data engineer, and built various high-throughput web services around said data. He has created new big data pipelines using Hadoop/Pig/MapReduce, and created machine learning models to predict click-through rate, news feed recommender systems using linear regression, Bayesian Bandits, and collaborative filtering and validated the results using A/B testing.
He has taught undergraduate and graduate students in data science, statistics, machine learning, algorithms, calculus, computer graphics, and physics for students attending universities such as Columbia University, NYU, Humber College, and The New School.
Multiple businesses have benefitted from his web programming expertise. He does all the backend (server), frontend (HTML/JS/CSS), and operations/deployment work. Some of the technologies he has used are: Python, Ruby/Rails, PHP, Bootstrap, jQuery (Javascript), Backbone, and Angular. For storage/databases he has used MySQL, Postgres, Redis, MongoDB, and more.
February 23, 2017 / by Marco / Advanced, Artificial, Bundle, Business', deals, Deep, design, entrepreneur, guide, intelligence, Learning, website
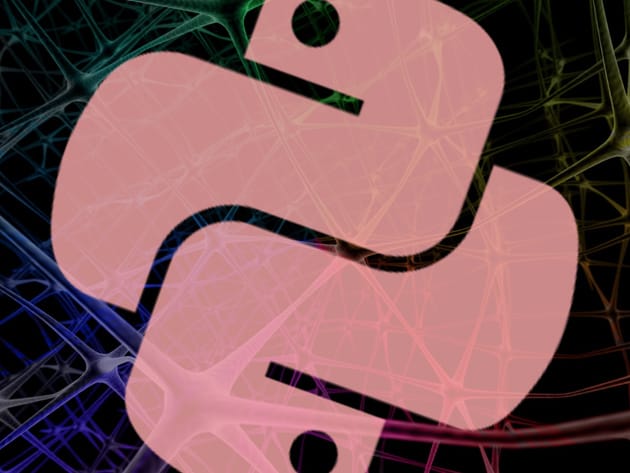
The Advanced Guide to Deep Learning and Artificial Intelligence Bundle for $42
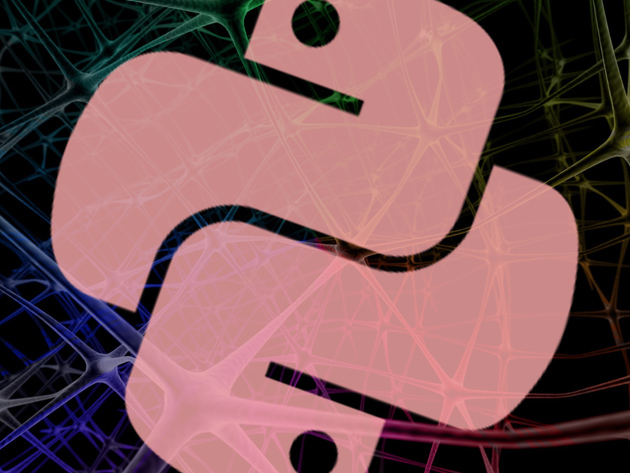
KEY FEATURES
In this course, intended to expand upon your knowledge of neural networks and deep learning, you’ll harness these concepts for computer vision using convolutional neural networks. Going in-depth on the concept of convolution, you’ll discover its wide range of applications, from generating image effects to modeling artificial organs.
- Access 25 lectures & 3 hours of content 24/7
- Explore the StreetView House Number (SVHN) dataset using convolutional neural networks (CNNs)
- Build convolutional filters that can be applied to audio or imaging
- Extend deep neural networks w/ just a few functions
- Test CNNs written in both Theano & TensorFlow
Note: we strongly recommend taking The Deep Learning & Artificial Intelligence Introductory Bundle before this course.
PRODUCT SPECS
Details & Requirements
- Length of time users can access this course: lifetime
- Access options: web streaming, mobile streaming
- Certification of completion not included
- Redemption deadline: redeem your code within 30 days of purchase
- Experience level required: all levels, but you must have some knowledge of calculus, linear algebra, probability, Python, Numpy, and be able to write a feedforward neural network in Theano and TensorFlow.
- All code for this course is available for download here, in the directory cnn_class
Compatibility
- Internet required
THE EXPERT
The Lazy Programmer is a data scientist, big data engineer, and full stack software engineer. For his master’s thesis he worked on brain-computer interfaces using machine learning. These assist non-verbal and non-mobile persons to communicate with their family and caregivers.
He has worked in online advertising and digital media as both a data scientist and big data engineer, and built various high-throughput web services around said data. He has created new big data pipelines using Hadoop/Pig/MapReduce, and created machine learning models to predict click-through rate, news feed recommender systems using linear regression, Bayesian Bandits, and collaborative filtering and validated the results using A/B testing.
He has taught undergraduate and graduate students in data science, statistics, machine learning, algorithms, calculus, computer graphics, and physics for students attending universities such as Columbia University, NYU, Humber College, and The New School.
Multiple businesses have benefitted from his web programming expertise. He does all the backend (server), frontend (HTML/JS/CSS), and operations/deployment work. Some of the technologies he has used are: Python, Ruby/Rails, PHP, Bootstrap, jQuery (Javascript), Backbone, and Angular. For storage/databases he has used MySQL, Postgres, Redis, MongoDB, and more.
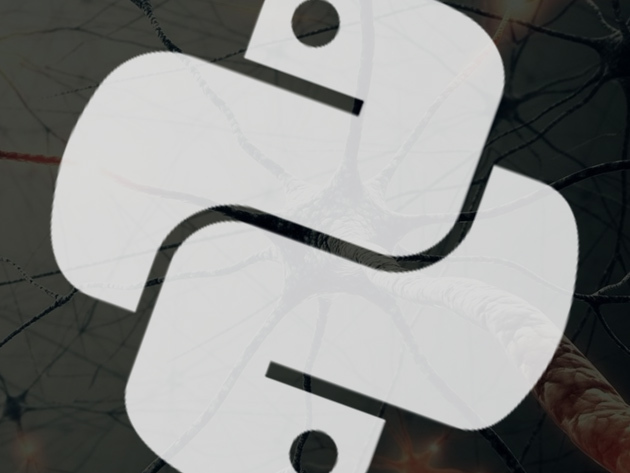
KEY FEATURES
In this course, you’ll dig deep into deep learning, discussing principal components analysis and a popular nonlinear dimensionality reduction technique known as t-distributed stochastic neighbor embedding (t-SNE). From there you’ll learn about a special type of unsupervised neural network called the autoencoder, understanding how to link many together to get a better performance out of deep neural networks.
- Access 30 lectures & 3 hours of content 24/7
- Discuss restricted Boltzmann machines (RBMs) & how to pretrain supervised deep neural networks
- Learn about Gibbs sampling
- Use PCA & t-SNE on features learned by autoencoders & RBMs
- Understand the most modern deep learning developments
PRODUCT SPECS
Details & Requirements
- Length of time users can access this course: lifetime
- Access options: web streaming, mobile streaming
- Certification of completion not included
- Redemption deadline: redeem your code within 30 days of purchase
- Experience level required: intermediate, but you must have some knowledge of calculus, linear algebra, probability, Python, Numpy, and be able to write a feedforward neural network in Theano and TensorFlow.
- All code for this course is available for download here, in the directory unsupervised_class2
Compatibility
- Internet required
THE EXPERT
The Lazy Programmer is a data scientist, big data engineer, and full stack software engineer. For his master’s thesis he worked on brain-computer interfaces using machine learning. These assist non-verbal and non-mobile persons to communicate with their family and caregivers.
He has worked in online advertising and digital media as both a data scientist and big data engineer, and built various high-throughput web services around said data. He has created new big data pipelines using Hadoop/Pig/MapReduce, and created machine learning models to predict click-through rate, news feed recommender systems using linear regression, Bayesian Bandits, and collaborative filtering and validated the results using A/B testing.
He has taught undergraduate and graduate students in data science, statistics, machine learning, algorithms, calculus, computer graphics, and physics for students attending universities such as Columbia University, NYU, Humber College, and The New School.
Multiple businesses have benefitted from his web programming expertise. He does all the backend (server), frontend (HTML/JS/CSS), and operations/deployment work. Some of the technologies he has used are: Python, Ruby/Rails, PHP, Bootstrap, jQuery (Javascript), Backbone, and Angular. For storage/databases he has used MySQL, Postgres, Redis, MongoDB, and more.
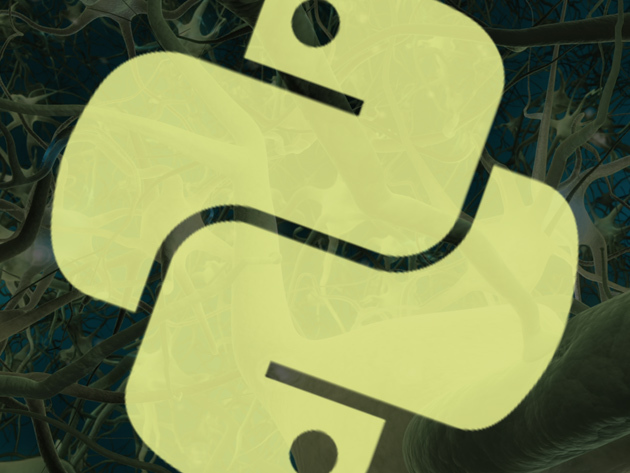
KEY FEATURES
A recurrent neural network is a class of artificial neural network where connections form a directed cycle, using their internal memory to process arbitrary sequences of inputs. This makes them capable of tasks like handwriting and speech recognition. In this course, you’ll explore this extremely expressive facet of deep learning and get up to speed on this revolutionary new advance.
- Access 32 lectures & 4 hours of content 24/7
- Get introduced to the Simple Recurrent Unit, also known as the Elman unit
- Extend the XOR problem as a parity problem
- Explore language modeling
- Learn Word2Vec to create word vectors or word embeddings
- Look at the long short-term memory unit (LSTM), & gated recurrent unit (GRU)
- Apply what you learn to practical problems like learning a language model from Wikipedia data
PRODUCT SPECS
Details & Requirements
- Length of time users can access this course: lifetime
- Access options: web streaming, mobile streaming
- Certification of completion not included
- Redemption deadline: redeem your code within 30 days of purchase
- Experience level required: all levels, but you must have some knowledge of calculus, linear algebra, probability, Python, Numpy, and be able to write a feedforward neural network in Theano and TensorFlow.
- All code for this course is available for download here, in the directory rnn_class
Compatibility
- Internet required
THE EXPERT
The Lazy Programmer is a data scientist, big data engineer, and full stack software engineer. For his master’s thesis he worked on brain-computer interfaces using machine learning. These assist non-verbal and non-mobile persons to communicate with their family and caregivers.
He has worked in online advertising and digital media as both a data scientist and big data engineer, and built various high-throughput web services around said data. He has created new big data pipelines using Hadoop/Pig/MapReduce, and created machine learning models to predict click-through rate, news feed recommender systems using linear regression, Bayesian Bandits, and collaborative filtering and validated the results using A/B testing.
He has taught undergraduate and graduate students in data science, statistics, machine learning, algorithms, calculus, computer graphics, and physics for students attending universities such as Columbia University, NYU, Humber College, and The New School.
Multiple businesses have benefitted from his web programming expertise. He does all the backend (server), frontend (HTML/JS/CSS), and operations/deployment work. Some of the technologies he has used are: Python, Ruby/Rails, PHP, Bootstrap, jQuery (Javascript), Backbone, and Angular. For storage/databases he has used MySQL, Postgres, Redis, MongoDB, and more.
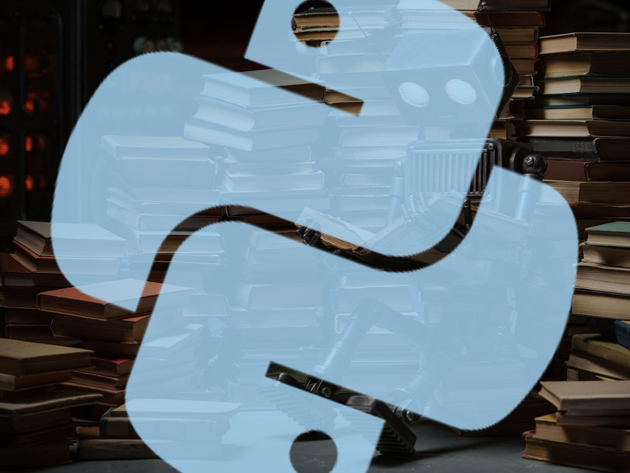
KEY FEATURES
In this course you’ll explore advanced natural language processing – the field of computer science and AI that concerns interactions between computer and human languages. Over the course you’ll learn four new NLP architectures and explore classic NLP problems like parts-of-speech tagging and named entity recognition, and use recurrent neural networks to solve them. By course’s end, you’ll have a firm grasp on natural language processing and its many applications.
- Access 40 lectures & 4.5 hours of content 24/7
- Discover Word2Vec & how it maps words to a vector space
- Explore GLoVe’s use of matrix factorization & how it contributes to recommendation systems
- Learn about recursive neural networks which will help solve the problem of negation in sentiment analysis
PRODUCT SPECS
Details & Requirements
- Length of time users can access this course: lifetime
- Access options: web streaming, mobile streaming
- Certification of completion not included
- Redemption deadline: redeem your code within 30 days of purchase
- Experience level required: advanced, but you must have some knowledge of calculus, linear algebra, probability, Python, Numpy, and be able to write a feedforward neural network in Theano and TensorFlow.
- All code for this course is available for download here, in the directory nlp_class2
Compatibility
- Internet required
THE EXPERT
The Lazy Programmer is a data scientist, big data engineer, and full stack software engineer. For his master’s thesis he worked on brain-computer interfaces using machine learning. These assist non-verbal and non-mobile persons to communicate with their family and caregivers.
He has worked in online advertising and digital media as both a data scientist and big data engineer, and built various high-throughput web services around said data. He has created new big data pipelines using Hadoop/Pig/MapReduce, and created machine learning models to predict click-through rate, news feed recommender systems using linear regression, Bayesian Bandits, and collaborative filtering and validated the results using A/B testing.
He has taught undergraduate and graduate students in data science, statistics, machine learning, algorithms, calculus, computer graphics, and physics for students attending universities such as Columbia University, NYU, Humber College, and The New School.
Multiple businesses have benefitted from his web programming expertise. He does all the backend (server), frontend (HTML/JS/CSS), and operations/deployment work. Some of the technologies he has used are: Python, Ruby/Rails, PHP, Bootstrap, jQuery (Javascript), Backbone, and Angular. For storage/databases he has used MySQL, Postgres, Redis, MongoDB, and more.
December 3, 2016 / by Marco / Artificial, Bundle, Business', deals, Deep, design, entrepreneur, intelligence, Introductory, Learning, website
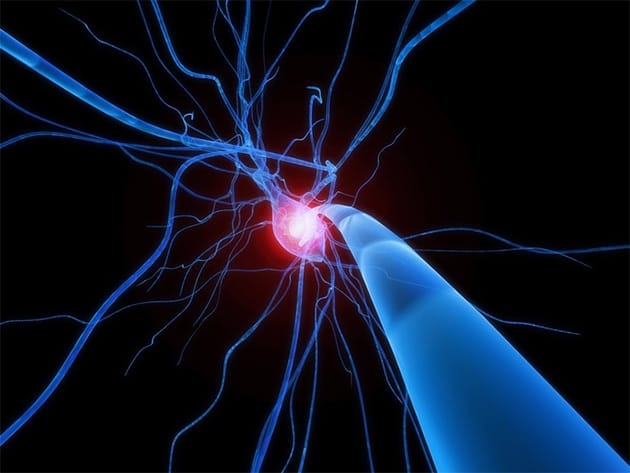
The Deep Learning and Artificial Intelligence Introductory Bundle for $39
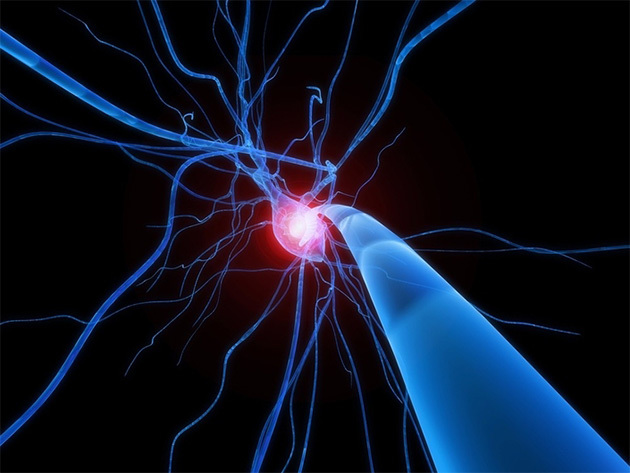
KEY FEATURES
Deep Learning is a set of powerful algorithms that are the force behind self-driving cars, image searching, voice recognition, and many, many more applications we consider decidedly “futuristic.” One of the central foundations of deep learning is linear regression; using probability theory to gain deeper insight into the “line of best fit.” This is the first step to building machines that, in effect, act like neurons in a neural network as they learn while they’re fed more information. In this course, you’ll start with the basics of building a linear regression module in Python, and progress into practical machine learning issues that will provide the foundations for an exploration of Deep Learning.
- Access 20 lectures & 2 hours of content 24/7
- Use a 1-D linear regression to prove Moore’s Law
- Learn how to create a machine learning model that can learn from multiple inputs
- Apply multi-dimensional linear regression to predict a patient’s systolic blood pressure given their age & weight
- Discuss generalization, overfitting, train-test splits, & other issues that may arise while performing data analysis
Like what you’re learning? Try out the The Advanced Guide to Deep Learning and Artificial Intelligence next.
PRODUCT SPECS
Details & Requirements
- Length of time users can access this course: lifetime
- Access options: web streaming, mobile streaming
- Certification of completion not included
- Redemption deadline: redeem your code within 30 days of purchase
- Experience level required: all levels, but you must have some knowledge of calculus, linear algebra, probability, Python, and Numpy
- All code for this course is available for download here, in the directory linear_regression_class
Compatibility
- Internet required
THE EXPERT
The Lazy Programmer is a data scientist, big data engineer, and full stack software engineer. For his master’s thesis he worked on brain-computer interfaces using machine learning. These assist non-verbal and non-mobile persons to communicate with their family and caregivers.
He has worked in online advertising and digital media as both a data scientist and big data engineer, and built various high-throughput web services around said data. He has created new big data pipelines using Hadoop/Pig/MapReduce, and created machine learning models to predict click-through rate, news feed recommender systems using linear regression, Bayesian Bandits, and collaborative filtering and validated the results using A/B testing.
He has taught undergraduate and graduate students in data science, statistics, machine learning, algorithms, calculus, computer graphics, and physics for students attending universities such as Columbia University, NYU, Humber College, and The New School.
Multiple businesses have benefitted from his web programming expertise. He does all the backend (server), frontend (HTML/JS/CSS), and operations/deployment work. Some of the technologies he has used are: Python, Ruby/Rails, PHP, Bootstrap, jQuery (Javascript), Backbone, and Angular. For storage/databases he has used MySQL, Postgres, Redis, MongoDB, and more.
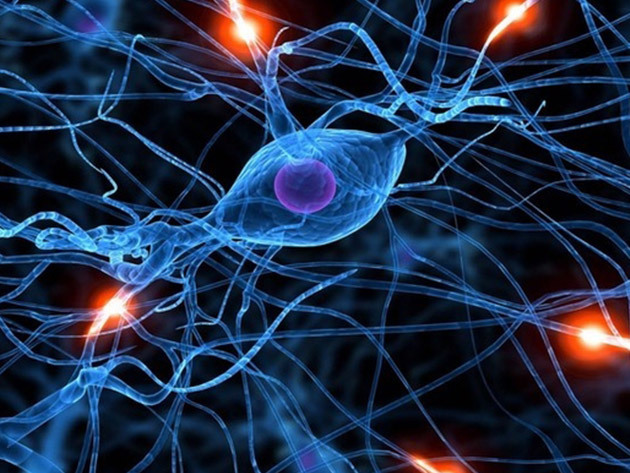
KEY FEATURES
Logistic regression is one of the most fundamental techniques used in machine learning, data science, and statistics, as it may be used to create a classification or labeling algorithm that quite resembles a biological neuron. Logistic regression units, by extension, are the basic bricks in the neural network, the central architecture in deep learning. In this course, you’ll come to terms with logistic regression using practical, real-world examples to fully appreciate the vast applications of Deep Learning.
- Access 31 lectures & 3 hours of content 24/7
- Code your own logistic regression module in Python
- Complete a course project that predicts user actions on a website given user data
- Use Deep Learning for facial expression recognition
- Understand how to make data-driven decisions
Like what you’re learning? Try out the The Advanced Guide to Deep Learning and Artificial Intelligence next.
PRODUCT SPECS
Details & Requirements
- Length of time users can access this course: lifetime
- Access options: web streaming, mobile streaming
- Certification of completion not included
- Redemption deadline: redeem your code within 30 days of purchase
- Experience level required: all levels, but you must have some knowledge of calculus, linear algebra, probability, Python, and Numpy
- All code for this course is available for download here, in the directory logistic_regression_class
Compatibility
- Internet required
THE EXPERT
The Lazy Programmer is a data scientist, big data engineer, and full stack software engineer. For his master’s thesis he worked on brain-computer interfaces using machine learning. These assist non-verbal and non-mobile persons to communicate with their family and caregivers.
He has worked in online advertising and digital media as both a data scientist and big data engineer, and built various high-throughput web services around said data. He has created new big data pipelines using Hadoop/Pig/MapReduce, and created machine learning models to predict click-through rate, news feed recommender systems using linear regression, Bayesian Bandits, and collaborative filtering and validated the results using A/B testing.
He has taught undergraduate and graduate students in data science, statistics, machine learning, algorithms, calculus, computer graphics, and physics for students attending universities such as Columbia University, NYU, Humber College, and The New School.
Multiple businesses have benefitted from his web programming expertise. He does all the backend (server), frontend (HTML/JS/CSS), and operations/deployment work. Some of the technologies he has used are: Python, Ruby/Rails, PHP, Bootstrap, jQuery (Javascript), Backbone, and Angular. For storage/databases he has used MySQL, Postgres, Redis, MongoDB, and more.
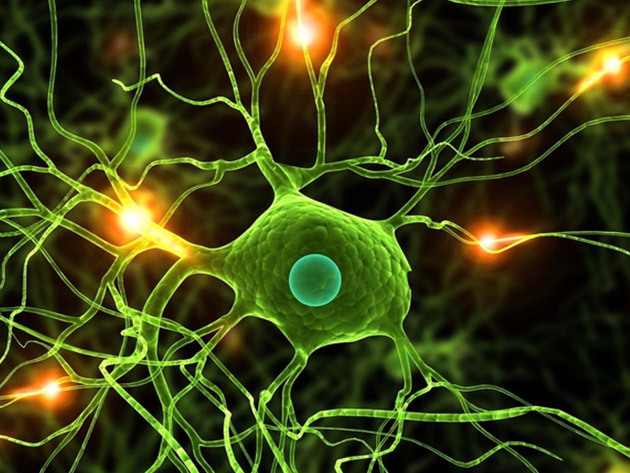
KEY FEATURES
Artificial neural networks are the architecture that make Apple’s Siri recognize your voice, Tesla’s self-driving cars know where to turn, Google Translate learn new languages, and so many more technological features you have quite possibly taken for granted. The data science that unites all of them is Deep Learning. In this course, you’ll build your very first neural network, going beyond basic models to build networks that automatically learn features.
- Access 37 lectures & 4 hours of content 24/7
- Extend the binary classification model to multiple classes uing the softmax function
- Code the important training method, backpropagation, in Numpy
- Implement a neural network using Google’s TensorFlow library
- Predict user actions on a website given user data using a neural network
- Use Deep Learning for facial expression recognition
- Learn some of the newest development in neural networks
Like what you’re learning? Try out the The Advanced Guide to Deep Learning and Artificial Intelligence next.
PRODUCT SPECS
Details & Requirements
- Length of time users can access this course: lifetime
- Access options: web streaming, mobile streaming
- Certification of completion not included
- Redemption deadline: redeem your code within 30 days of purchase
- Experience level required: intermediate, but you must have some knowledge of calculus, linear algebra, probability, Python, and Numpy
- All code for this course is available for download here, in the directory ann_class
Compatibility
- Internet required
THE EXPERT
The Lazy Programmer is a data scientist, big data engineer, and full stack software engineer. For his master’s thesis he worked on brain-computer interfaces using machine learning. These assist non-verbal and non-mobile persons to communicate with their family and caregivers.
He has worked in online advertising and digital media as both a data scientist and big data engineer, and built various high-throughput web services around said data. He has created new big data pipelines using Hadoop/Pig/MapReduce, and created machine learning models to predict click-through rate, news feed recommender systems using linear regression, Bayesian Bandits, and collaborative filtering and validated the results using A/B testing.
He has taught undergraduate and graduate students in data science, statistics, machine learning, algorithms, calculus, computer graphics, and physics for students attending universities such as Columbia University, NYU, Humber College, and The New School.
Multiple businesses have benefitted from his web programming expertise. He does all the backend (server), frontend (HTML/JS/CSS), and operations/deployment work. Some of the technologies he has used are: Python, Ruby/Rails, PHP, Bootstrap, jQuery (Javascript), Backbone, and Angular. For storage/databases he has used MySQL, Postgres, Redis, MongoDB, and more.
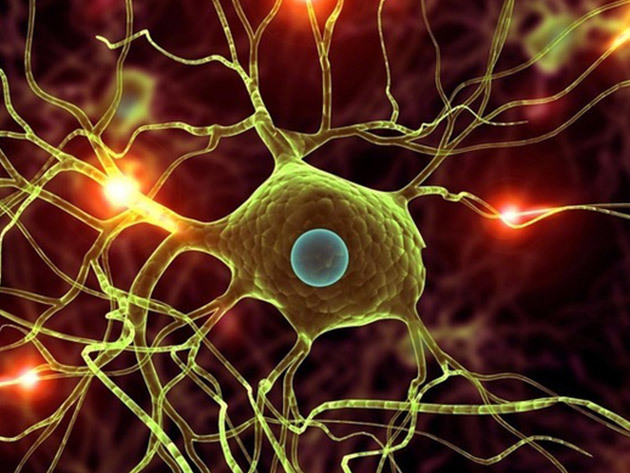
KEY FEATURES
The applications of Deep Learning are many, and constantly growing, just like the neural networks that it supports. In this course, you’ll delve into advanced concepts of Deep Learning, starting with the basics of TensorFlow and Theano, understanding how to build neural networks with these popular tools. Using these tools, you’ll learn how to build and understand a neural network, knowing exactly how to visualize what is happening within a model as it learns.
- Access 23 lectures & 3 hours of programming 24/7
- Discover batch & stochastic gradient descent, two techniques that allow you to train on a small sample of data at each iteration, greatly speeding up training time
- Discuss how momentum can carry you through local minima
- Learn adaptive learning rate techniques like AdaGrad & RMSprop
- Explore dropout regularization & other modern neural network techniques
- Understand the variables & expressions of TensorFlow & Theano
- Set up a GPU-instance on AWS & compare the speed of CPU vs GPU for training a deep neural network
- Look at the MNIST dataset & compare against known benchmarks
Like what you’re learning? Try out the The Advanced Guide to Deep Learning and Artificial Intelligence next.
PRODUCT SPECS
Details & Requirements
- Length of time users can access this course: lifetime
- Access options: web streaming, mobile streaming
- Certification of completion not included
- Redemption deadline: redeem your code within 30 days of purchase
- Experience level required: all levels, but you must have some knowledge of calculus, linear algebra, probability, Python, and Numpy
- All code for this course is available for download here, in the directory ann_class2
Compatibility
- Internet required
THE EXPERT
The Lazy Programmer is a data scientist, big data engineer, and full stack software engineer. For his master’s thesis he worked on brain-computer interfaces using machine learning. These assist non-verbal and non-mobile persons to communicate with their family and caregivers.
He has worked in online advertising and digital media as both a data scientist and big data engineer, and built various high-throughput web services around said data. He has created new big data pipelines using Hadoop/Pig/MapReduce, and created machine learning models to predict click-through rate, news feed recommender systems using linear regression, Bayesian Bandits, and collaborative filtering and validated the results using A/B testing.
He has taught undergraduate and graduate students in data science, statistics, machine learning, algorithms, calculus, computer graphics, and physics for students attending universities such as Columbia University, NYU, Humber College, and The New School.
Multiple businesses have benefitted from his web programming expertise. He does all the backend (server), frontend (HTML/JS/CSS), and operations/deployment work. Some of the technologies he has used are: Python, Ruby/Rails, PHP, Bootstrap, jQuery (Javascript), Backbone, and Angular. For storage/databases he has used MySQL, Postgres, Redis, MongoDB, and more.
November 29, 2016 / by Marco / Advanced, Artificial, Business', deals, Deep, design, entrepreneur, guide, intelligence, Learning, website
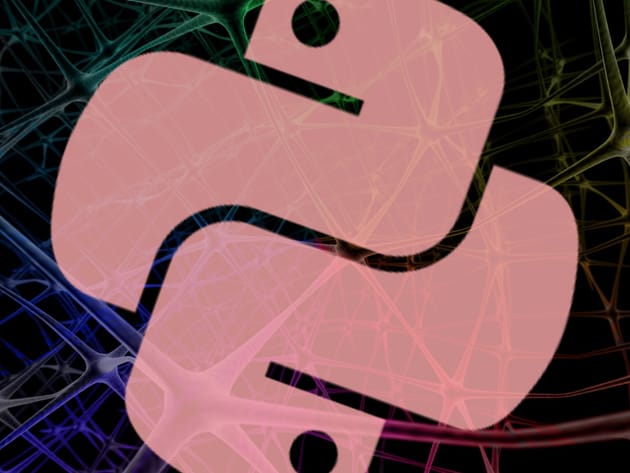
The Advanced Guide to Deep Learning and Artificial Intelligence for $42
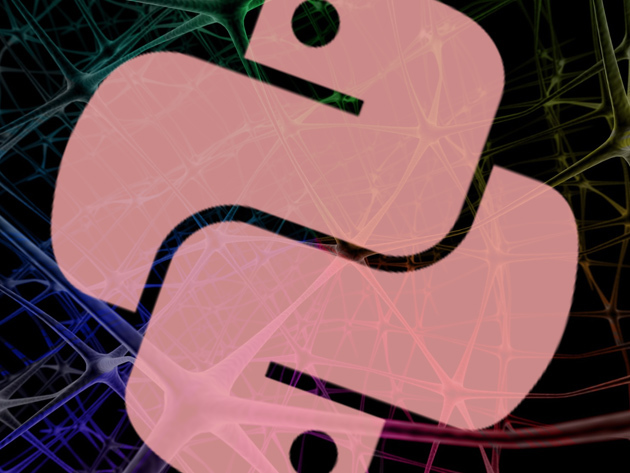
KEY FEATURES
In this course, intended to expand upon your knowledge of neural networks and deep learning, you’ll harness these concepts for computer vision using convolutional neural networks. Going in-depth on the concept of convolution, you’ll discover its wide range of applications, from generating image effects to modeling artificial organs.
- Access 25 lectures & 3 hours of content 24/7
- Explore the StreetView House Number (SVHN) dataset using convolutional neural networks (CNNs)
- Build convolutional filters that can be applied to audio or imaging
- Extend deep neural networks w/ just a few functions
- Test CNNs written in both Theano & TensorFlow
Note: we strongly recommend taking The Deep Learning & Artificial Intelligence Introductory Bundle before this course.
PRODUCT SPECS
Details & Requirements
- Length of time users can access this course: lifetime
- Access options: web streaming, mobile streaming
- Certification of completion not included
- Redemption deadline: redeem your code within 30 days of purchase
- Experience level required: all levels, but you must have some knowledge of calculus, linear algebra, probability, Python, Numpy, and be able to write a feedforward neural network in Theano and TensorFlow.
- All code for this course is available for download here, in the directory cnn_class
Compatibility
- Internet required
THE EXPERT
The Lazy Programmer is a data scientist, big data engineer, and full stack software engineer. For his master’s thesis he worked on brain-computer interfaces using machine learning. These assist non-verbal and non-mobile persons to communicate with their family and caregivers.
He has worked in online advertising and digital media as both a data scientist and big data engineer, and built various high-throughput web services around said data. He has created new big data pipelines using Hadoop/Pig/MapReduce, and created machine learning models to predict click-through rate, news feed recommender systems using linear regression, Bayesian Bandits, and collaborative filtering and validated the results using A/B testing.
He has taught undergraduate and graduate students in data science, statistics, machine learning, algorithms, calculus, computer graphics, and physics for students attending universities such as Columbia University, NYU, Humber College, and The New School.
Multiple businesses have benefitted from his web programming expertise. He does all the backend (server), frontend (HTML/JS/CSS), and operations/deployment work. Some of the technologies he has used are: Python, Ruby/Rails, PHP, Bootstrap, jQuery (Javascript), Backbone, and Angular. For storage/databases he has used MySQL, Postgres, Redis, MongoDB, and more.
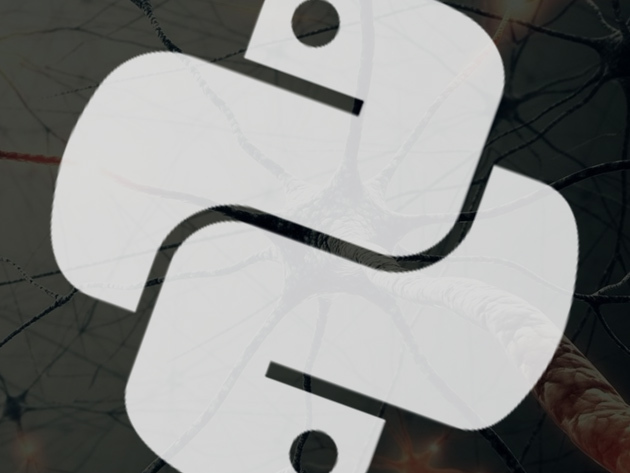
KEY FEATURES
In this course, you’ll dig deep into deep learning, discussing principal components analysis and a popular nonlinear dimensionality reduction technique known as t-distributed stochastic neighbor embedding (t-SNE). From there you’ll learn about a special type of unsupervised neural network called the autoencoder, understanding how to link many together to get a better performance out of deep neural networks.
- Access 30 lectures & 3 hours of content 24/7
- Discuss restricted Boltzmann machines (RBMs) & how to pretrain supervised deep neural networks
- Learn about Gibbs sampling
- Use PCA & t-SNE on features learned by autoencoders & RBMs
- Understand the most modern deep learning developments
PRODUCT SPECS
Details & Requirements
- Length of time users can access this course: lifetime
- Access options: web streaming, mobile streaming
- Certification of completion not included
- Redemption deadline: redeem your code within 30 days of purchase
- Experience level required: intermediate, but you must have some knowledge of calculus, linear algebra, probability, Python, Numpy, and be able to write a feedforward neural network in Theano and TensorFlow.
- All code for this course is available for download here, in the directory unsupervised_class2
Compatibility
- Internet required
THE EXPERT
The Lazy Programmer is a data scientist, big data engineer, and full stack software engineer. For his master’s thesis he worked on brain-computer interfaces using machine learning. These assist non-verbal and non-mobile persons to communicate with their family and caregivers.
He has worked in online advertising and digital media as both a data scientist and big data engineer, and built various high-throughput web services around said data. He has created new big data pipelines using Hadoop/Pig/MapReduce, and created machine learning models to predict click-through rate, news feed recommender systems using linear regression, Bayesian Bandits, and collaborative filtering and validated the results using A/B testing.
He has taught undergraduate and graduate students in data science, statistics, machine learning, algorithms, calculus, computer graphics, and physics for students attending universities such as Columbia University, NYU, Humber College, and The New School.
Multiple businesses have benefitted from his web programming expertise. He does all the backend (server), frontend (HTML/JS/CSS), and operations/deployment work. Some of the technologies he has used are: Python, Ruby/Rails, PHP, Bootstrap, jQuery (Javascript), Backbone, and Angular. For storage/databases he has used MySQL, Postgres, Redis, MongoDB, and more.
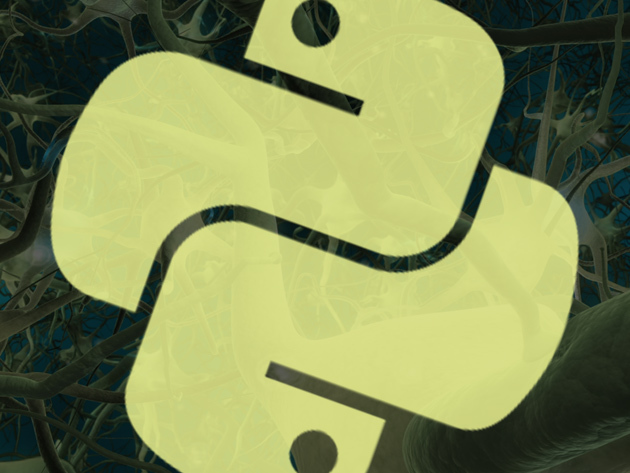
KEY FEATURES
A recurrent neural network is a class of artificial neural network where connections form a directed cycle, using their internal memory to process arbitrary sequences of inputs. This makes them capable of tasks like handwriting and speech recognition. In this course, you’ll explore this extremely expressive facet of deep learning and get up to speed on this revolutionary new advance.
- Access 32 lectures & 4 hours of content 24/7
- Get introduced to the Simple Recurrent Unit, also known as the Elman unit
- Extend the XOR problem as a parity problem
- Explore language modeling
- Learn Word2Vec to create word vectors or word embeddings
- Look at the long short-term memory unit (LSTM), & gated recurrent unit (GRU)
- Apply what you learn to practical problems like learning a language model from Wikipedia data
PRODUCT SPECS
Details & Requirements
- Length of time users can access this course: lifetime
- Access options: web streaming, mobile streaming
- Certification of completion not included
- Redemption deadline: redeem your code within 30 days of purchase
- Experience level required: all levels, but you must have some knowledge of calculus, linear algebra, probability, Python, Numpy, and be able to write a feedforward neural network in Theano and TensorFlow.
- All code for this course is available for download here, in the directory rnn_class
Compatibility
- Internet required
THE EXPERT
The Lazy Programmer is a data scientist, big data engineer, and full stack software engineer. For his master’s thesis he worked on brain-computer interfaces using machine learning. These assist non-verbal and non-mobile persons to communicate with their family and caregivers.
He has worked in online advertising and digital media as both a data scientist and big data engineer, and built various high-throughput web services around said data. He has created new big data pipelines using Hadoop/Pig/MapReduce, and created machine learning models to predict click-through rate, news feed recommender systems using linear regression, Bayesian Bandits, and collaborative filtering and validated the results using A/B testing.
He has taught undergraduate and graduate students in data science, statistics, machine learning, algorithms, calculus, computer graphics, and physics for students attending universities such as Columbia University, NYU, Humber College, and The New School.
Multiple businesses have benefitted from his web programming expertise. He does all the backend (server), frontend (HTML/JS/CSS), and operations/deployment work. Some of the technologies he has used are: Python, Ruby/Rails, PHP, Bootstrap, jQuery (Javascript), Backbone, and Angular. For storage/databases he has used MySQL, Postgres, Redis, MongoDB, and more.
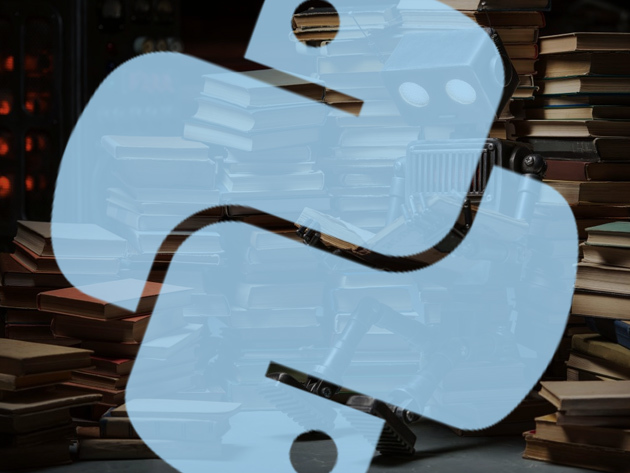
KEY FEATURES
In this course you’ll explore advanced natural language processing – the field of computer science and AI that concerns interactions between computer and human languages. Over the course you’ll learn four new NLP architectures and explore classic NLP problems like parts-of-speech tagging and named entity recognition, and use recurrent neural networks to solve them. By course’s end, you’ll have a firm grasp on natural language processing and its many applications.
- Access 40 lectures & 4.5 hours of content 24/7
- Discover Word2Vec & how it maps words to a vector space
- Explore GLoVe’s use of matrix factorization & how it contributes to recommendation systems
- Learn about recursive neural networks which will help solve the problem of negation in sentiment analysis
PRODUCT SPECS
Details & Requirements
- Length of time users can access this course: lifetime
- Access options: web streaming, mobile streaming
- Certification of completion not included
- Redemption deadline: redeem your code within 30 days of purchase
- Experience level required: advanced, but you must have some knowledge of calculus, linear algebra, probability, Python, Numpy, and be able to write a feedforward neural network in Theano and TensorFlow.
- All code for this course is available for download here, in the directory nlp_class2
Compatibility
- Internet required
THE EXPERT
The Lazy Programmer is a data scientist, big data engineer, and full stack software engineer. For his master’s thesis he worked on brain-computer interfaces using machine learning. These assist non-verbal and non-mobile persons to communicate with their family and caregivers.
He has worked in online advertising and digital media as both a data scientist and big data engineer, and built various high-throughput web services around said data. He has created new big data pipelines using Hadoop/Pig/MapReduce, and created machine learning models to predict click-through rate, news feed recommender systems using linear regression, Bayesian Bandits, and collaborative filtering and validated the results using A/B testing.
He has taught undergraduate and graduate students in data science, statistics, machine learning, algorithms, calculus, computer graphics, and physics for students attending universities such as Columbia University, NYU, Humber College, and The New School.
Multiple businesses have benefitted from his web programming expertise. He does all the backend (server), frontend (HTML/JS/CSS), and operations/deployment work. Some of the technologies he has used are: Python, Ruby/Rails, PHP, Bootstrap, jQuery (Javascript), Backbone, and Angular. For storage/databases he has used MySQL, Postgres, Redis, MongoDB, and more.
November 3, 2016 / by Marco / Business', deals, Deep, design, entrepreneur, Learning, Practical, TensorFlow, Theano, website
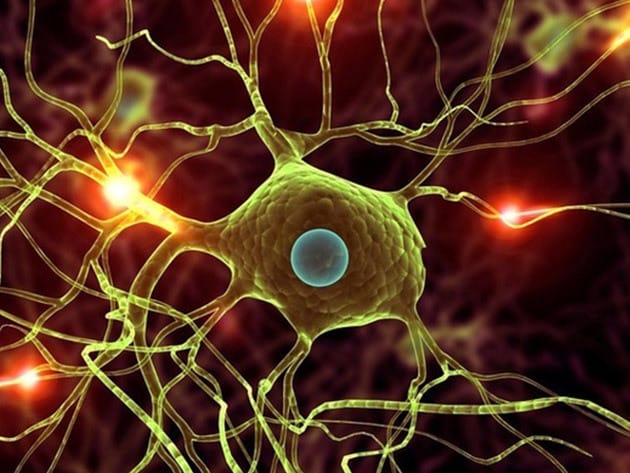
Practical Deep Learning in Theano and TensorFlow for $29
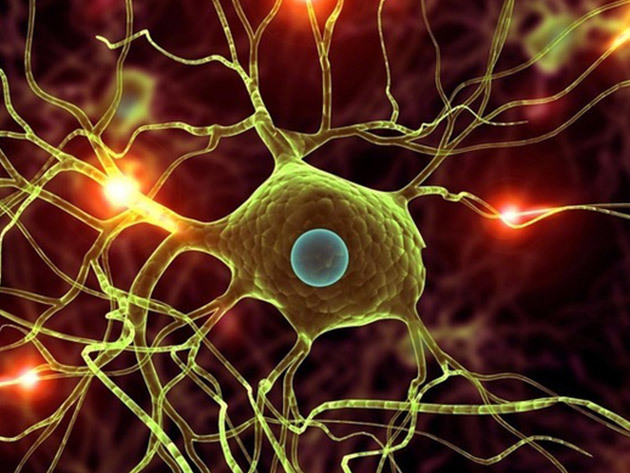
KEY FEATURES
The applications of Deep Learning are many, and constantly growing, just like the neural networks that it supports. In this course, you’ll delve into advanced concepts of Deep Learning, starting with the basics of TensorFlow and Theano, understanding how to build neural networks with these popular tools. Using these tools, you’ll learn how to build and understand a neural network, knowing exactly how to visualize what is happening within a model as it learns.
- Access 23 lectures & 3 hours of programming 24/7
- Discover batch & stochastic gradient descent, two techniques that allow you to train on a small sample of data at each iteration, greatly speeding up training time
- Discuss how momentum can carry you through local minima
- Learn adaptive learning rate techniques like AdaGrad & RMSprop
- Explore dropout regularization & other modern neural network techniques
- Understand the variables & expressions of TensorFlow & Theano
- Set up a GPU-instance on AWS & compare the speed of CPU vs GPU for training a deep neural network
- Look at the MNIST dataset & compare against known benchmarks
PRODUCT SPECS
Details & Requirements
- Length of time users can access this course: lifetime
- Access options: web streaming, mobile streaming
- Certification of completion not included
- Redemption deadline: redeem your code within 30 days of purchase
- Experience level required: all levels, but you must have some knowledge of calculus, linear algebra, probability, Python, and Numpy
- All code for this course is available for download here, in the directory ann_class2
Compatibility
- Internet required
THE EXPERT
The Lazy Programmer is a data scientist, big data engineer, and full stack software engineer. For his master’s thesis he worked on brain-computer interfaces using machine learning. These assist non-verbal and non-mobile persons to communicate with their family and caregivers.
He has worked in online advertising and digital media as both a data scientist and big data engineer, and built various high-throughput web services around said data. He has created new big data pipelines using Hadoop/Pig/MapReduce, and created machine learning models to predict click-through rate, news feed recommender systems using linear regression, Bayesian Bandits, and collaborative filtering and validated the results using A/B testing.
He has taught undergraduate and graduate students in data science, statistics, machine learning, algorithms, calculus, computer graphics, and physics for students attending universities such as Columbia University, NYU, Humber College, and The New School.
Multiple businesses have benefitted from his web programming expertise. He does all the backend (server), frontend (HTML/JS/CSS), and operations/deployment work. Some of the technologies he has used are: Python, Ruby/Rails, PHP, Bootstrap, jQuery (Javascript), Backbone, and Angular. For storage/databases he has used MySQL, Postgres, Redis, MongoDB, and more.
The Deep Learning & Artificial Intelligence Introductory Bundle for $39
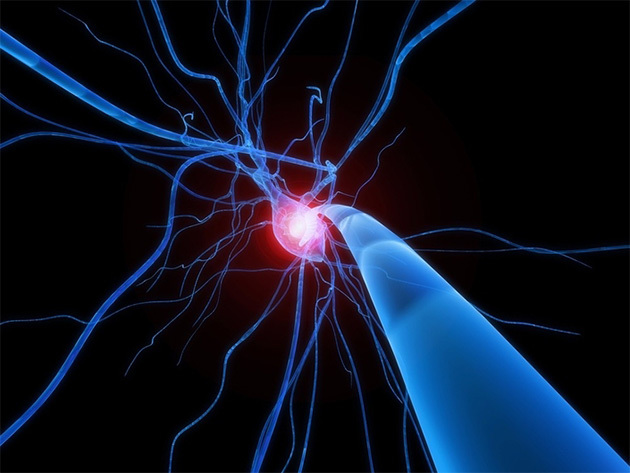
KEY FEATURES
Deep Learning is a set of powerful algorithms that are the force behind self-driving cars, image searching, voice recognition, and many, many more applications we consider decidedly “futuristic.” One of the central foundations of deep learning is linear regression; using probability theory to gain deeper insight into the “line of best fit.” This is the first step to building machines that, in effect, act like neurons in a neural network as they learn while they’re fed more information. In this course, you’ll start with the basics of building a linear regression module in Python, and progress into practical machine learning issues that will provide the foundations for an exploration of Deep Learning.
- Access 20 lectures & 2 hours of content 24/7
- Use a 1-D linear regression to prove Moore’s Law
- Learn how to create a machine learning model that can learn from multiple inputs
- Apply multi-dimensional linear regression to predict a patient’s systolic blood pressure given their age & weight
- Discuss generalization, overfitting, train-test splits, & other issues that may arise while performing data analysis
PRODUCT SPECS
Details & Requirements
- Length of time users can access this course: lifetime
- Access options: web streaming, mobile streaming
- Certification of completion not included
- Redemption deadline: redeem your code within 30 days of purchase
- Experience level required: all levels, but you must have some knowledge of calculus, linear algebra, probability, Python, and Numpy
- All code for this course is available for download here, in the directory linear_regression_class
Compatibility
- Internet required
THE EXPERT
The Lazy Programmer is a data scientist, big data engineer, and full stack software engineer. For his master’s thesis he worked on brain-computer interfaces using machine learning. These assist non-verbal and non-mobile persons to communicate with their family and caregivers.
He has worked in online advertising and digital media as both a data scientist and big data engineer, and built various high-throughput web services around said data. He has created new big data pipelines using Hadoop/Pig/MapReduce, and created machine learning models to predict click-through rate, news feed recommender systems using linear regression, Bayesian Bandits, and collaborative filtering and validated the results using A/B testing.
He has taught undergraduate and graduate students in data science, statistics, machine learning, algorithms, calculus, computer graphics, and physics for students attending universities such as Columbia University, NYU, Humber College, and The New School.
Multiple businesses have benefitted from his web programming expertise. He does all the backend (server), frontend (HTML/JS/CSS), and operations/deployment work. Some of the technologies he has used are: Python, Ruby/Rails, PHP, Bootstrap, jQuery (Javascript), Backbone, and Angular. For storage/databases he has used MySQL, Postgres, Redis, MongoDB, and more.
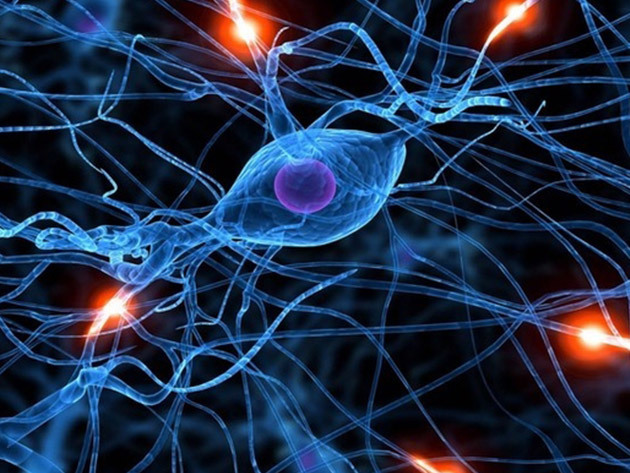
KEY FEATURES
Logistic regression is one of the most fundamental techniques used in machine learning, data science, and statistics, as it may be used to create a classification or labeling algorithm that quite resembles a biological neuron. Logistic regression units, by extension, are the basic bricks in the neural network, the central architecture in deep learning. In this course, you’ll come to terms with logistic regression using practical, real-world examples to fully appreciate the vast applications of Deep Learning.
- Access 31 lectures & 3 hours of content 24/7
- Code your own logistic regression module in Python
- Complete a course project that predicts user actions on a website given user data
- Use Deep Learning for facial expression recognition
- Understand how to make data-driven decisions
PRODUCT SPECS
Details & Requirements
- Length of time users can access this course: lifetime
- Access options: web streaming, mobile streaming
- Certification of completion not included
- Redemption deadline: redeem your code within 30 days of purchase
- Experience level required: all levels, but you must have some knowledge of calculus, linear algebra, probability, Python, and Numpy
- All code for this course is available for download here, in the directory logistic_regression_class
Compatibility
- Internet required
THE EXPERT
The Lazy Programmer is a data scientist, big data engineer, and full stack software engineer. For his master’s thesis he worked on brain-computer interfaces using machine learning. These assist non-verbal and non-mobile persons to communicate with their family and caregivers.
He has worked in online advertising and digital media as both a data scientist and big data engineer, and built various high-throughput web services around said data. He has created new big data pipelines using Hadoop/Pig/MapReduce, and created machine learning models to predict click-through rate, news feed recommender systems using linear regression, Bayesian Bandits, and collaborative filtering and validated the results using A/B testing.
He has taught undergraduate and graduate students in data science, statistics, machine learning, algorithms, calculus, computer graphics, and physics for students attending universities such as Columbia University, NYU, Humber College, and The New School.
Multiple businesses have benefitted from his web programming expertise. He does all the backend (server), frontend (HTML/JS/CSS), and operations/deployment work. Some of the technologies he has used are: Python, Ruby/Rails, PHP, Bootstrap, jQuery (Javascript), Backbone, and Angular. For storage/databases he has used MySQL, Postgres, Redis, MongoDB, and more.
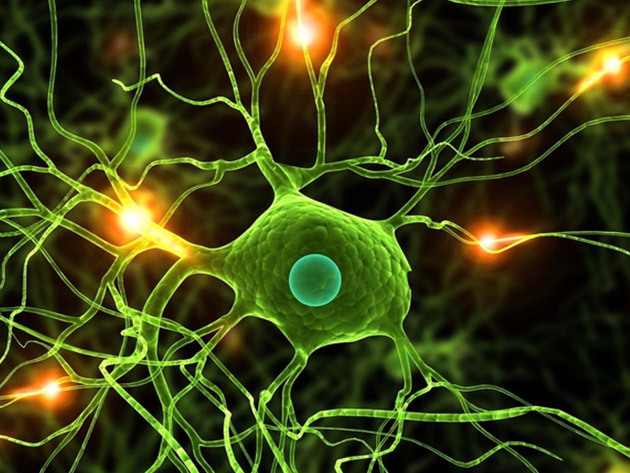
KEY FEATURES
Artificial neural networks are the architecture that make Apple’s Siri recognize your voice, Tesla’s self-driving cars know where to turn, Google Translate learn new languages, and so many more technological features you have quite possibly taken for granted. The data science that unites all of them is Deep Learning. In this course, you’ll build your very first neural network, going beyond basic models to build networks that automatically learn features.
- Access 37 lectures & 4 hours of content 24/7
- Extend the binary classification model to multiple classes uing the softmax function
- Code the important training method, backpropagation, in Numpy
- Implement a neural network using Google’s TensorFlow library
- Predict user actions on a website given user data using a neural network
- Use Deep Learning for facial expression recognition
- Learn some of the newest development in neural networks
PRODUCT SPECS
Details & Requirements
- Length of time users can access this course: lifetime
- Access options: web streaming, mobile streaming
- Certification of completion not included
- Redemption deadline: redeem your code within 30 days of purchase
- Experience level required: intermediate, but you must have some knowledge of calculus, linear algebra, probability, Python, and Numpy
- All code for this course is available for download here, in the directory ann_class
Compatibility
- Internet required
THE EXPERT
The Lazy Programmer is a data scientist, big data engineer, and full stack software engineer. For his master’s thesis he worked on brain-computer interfaces using machine learning. These assist non-verbal and non-mobile persons to communicate with their family and caregivers.
He has worked in online advertising and digital media as both a data scientist and big data engineer, and built various high-throughput web services around said data. He has created new big data pipelines using Hadoop/Pig/MapReduce, and created machine learning models to predict click-through rate, news feed recommender systems using linear regression, Bayesian Bandits, and collaborative filtering and validated the results using A/B testing.
He has taught undergraduate and graduate students in data science, statistics, machine learning, algorithms, calculus, computer graphics, and physics for students attending universities such as Columbia University, NYU, Humber College, and The New School.
Multiple businesses have benefitted from his web programming expertise. He does all the backend (server), frontend (HTML/JS/CSS), and operations/deployment work. Some of the technologies he has used are: Python, Ruby/Rails, PHP, Bootstrap, jQuery (Javascript), Backbone, and Angular. For storage/databases he has used MySQL, Postgres, Redis, MongoDB, and more.
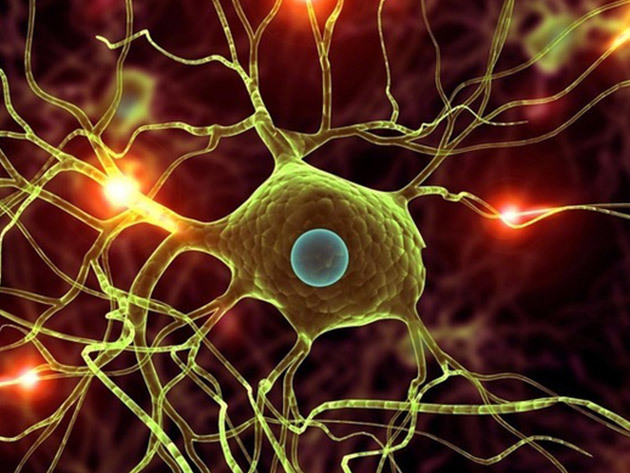
KEY FEATURES
The applications of Deep Learning are many, and constantly growing, just like the neural networks that it supports. In this course, you’ll delve into advanced concepts of Deep Learning, starting with the basics of TensorFlow and Theano, understanding how to build neural networks with these popular tools. Using these tools, you’ll learn how to build and understand a neural network, knowing exactly how to visualize what is happening within a model as it learns.
- Access 23 lectures & 3 hours of programming 24/7
- Discover batch & stochastic gradient descent, two techniques that allow you to train on a small sample of data at each iteration, greatly speeding up training time
- Discuss how momentum can carry you through local minima
- Learn adaptive learning rate techniques like AdaGrad & RMSprop
- Explore dropout regularization & other modern neural network techniques
- Understand the variables & expressions of TensorFlow & Theano
- Set up a GPU-instance on AWS & compare the speed of CPU vs GPU for training a deep neural network
- Look at the MNIST dataset & compare against known benchmarks
PRODUCT SPECS
Details & Requirements
- Length of time users can access this course: lifetime
- Access options: web streaming, mobile streaming
- Certification of completion not included
- Redemption deadline: redeem your code within 30 days of purchase
- Experience level required: all levels, but you must have some knowledge of calculus, linear algebra, probability, Python, and Numpy
- All code for this course is available for download here, in the directory ann_class2
Compatibility
- Internet required
THE EXPERT
The Lazy Programmer is a data scientist, big data engineer, and full stack software engineer. For his master’s thesis he worked on brain-computer interfaces using machine learning. These assist non-verbal and non-mobile persons to communicate with their family and caregivers.
He has worked in online advertising and digital media as both a data scientist and big data engineer, and built various high-throughput web services around said data. He has created new big data pipelines using Hadoop/Pig/MapReduce, and created machine learning models to predict click-through rate, news feed recommender systems using linear regression, Bayesian Bandits, and collaborative filtering and validated the results using A/B testing.
He has taught undergraduate and graduate students in data science, statistics, machine learning, algorithms, calculus, computer graphics, and physics for students attending universities such as Columbia University, NYU, Humber College, and The New School.
Multiple businesses have benefitted from his web programming expertise. He does all the backend (server), frontend (HTML/JS/CSS), and operations/deployment work. Some of the technologies he has used are: Python, Ruby/Rails, PHP, Bootstrap, jQuery (Javascript), Backbone, and Angular. For storage/databases he has used MySQL, Postgres, Redis, MongoDB, and more.
- 1
- 2